Artificial Intelligence to optimize CT
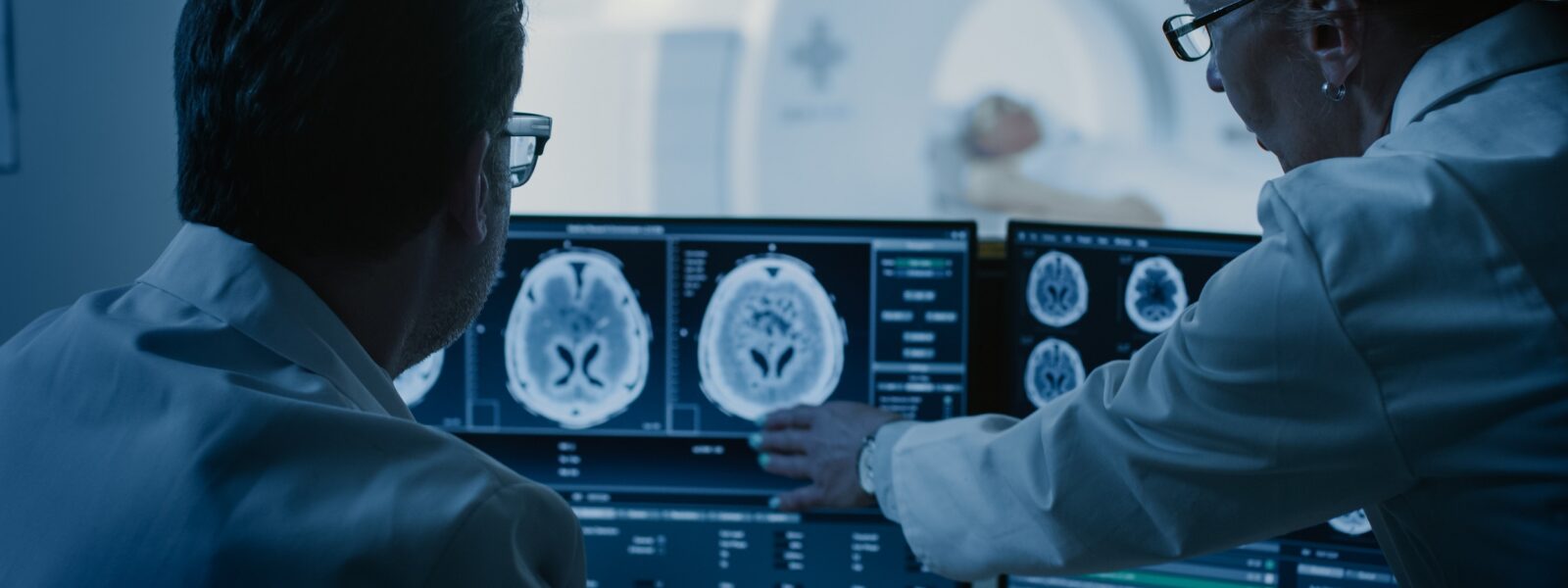
A group of researchers, medical physicists and radiologists from the Department of Physics and Astronomy of the University of Florence, the Careggi University Hospital, and the Azienda Usl Toscana centro, led by Dr. Sandra Doria of the Institute of Chemistry of Organo-Metallic compounds of the National Research Council in Florence (CNR-Iccom), has succeeded in automating the process of assessing image quality in computed tomography (CT) examinations using artificial intelligence, in order to reduce radiation to the patient. The project, whose method was described in a study published in the Journal of Medical Imaging (JMI), was also carried out by the Istituto Superiore di Sanità and the Fondazione Bruno Kessler of Trento, using computational resources provided by Uniser Pistoia.
Computed tomography is one of the most powerful and consolidated diagnostic tools available to modern medicine. However, manual analysis of the images that are produced through this methodology is time-consuming, and their quality is directly proportional to the amount of X-ray radiation a patient must undergo for the purpose. “Our group created an algorithm by analyzing the data generated by visual examination that several radiologists performed on Tc images of a puppet, made for the purpose of replicating the characteristics of human tissues and the presence of artificial injuries. Then two artificial intelligence models were developed, which were trained and tested through the use of the images and doctors’ responses previously collected,” explains Sandra Doria (CNR-Iccom), research coordinator.
These models could represent a strategy for automatically assessing the quality of a Tc image, which will enable optimization of radiation dosage so that patients are not exposed to excessive amounts of X-rays. “During treatments or diagnostic procedures, a patient should be exposed to minimal levels of radiation, according to the principle known as ‘as low as reasonably achievable’ (ALARA). With this in mind, the medical staff must find a trade-off between X-ray exposure and obtaining good quality images, also to avoid misdiagnosis. The results we have obtained through this study are very promising: our models can accurately identify an object placed in the puppet, as a radiologist would be able to do. We hope, in the near future, to be able to apply these models on a larger scale and make evaluations even faster and safer, thus greatly simplifying the process of optimizing the radiation dose used in CT protocols. This is crucial for reducing health risks for patients and optimizing the timing of medical evaluations,” Doria concluded.
One difficulty we encountered,” adds Diego Sona, FBK researcher and co-author of the study, “and one that raises some considerations is that the models we developed, which are the result of an interdisciplinary collaboration among physicists, doctors and computer scientists, tended to succeed better than radiologists in detecting anomalies in images. Therefore, to achieve a similar behavior to that of expert radiologists’, we had to limit the computational power of the models. This suggests further research scenarios on reducing radiation dosage but also on supporting radiologists in image analysis.”